Lead image courtesy of Getty Images
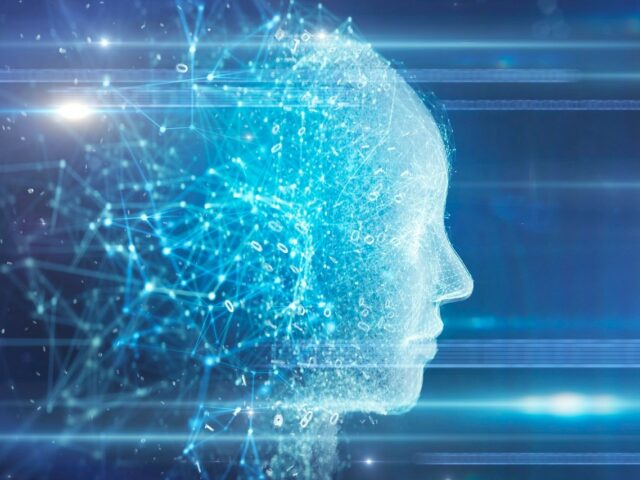
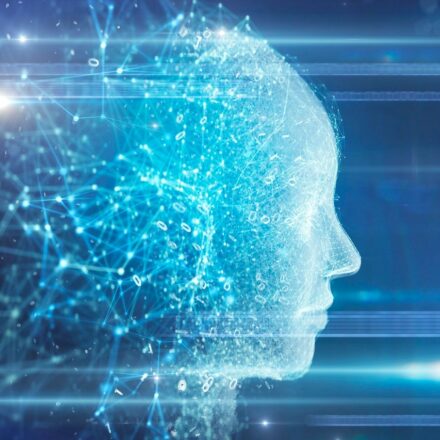
Security, Trust and Ethics
Building ethical AI goes beyond computing
The actual work of rooting out bias from imperfect systems will have to come from humans, not machines.
Lead image courtesy of Getty Images
Artificial intelligence (AI) is neither inherently good nor bad. Therefore, the shortcomings of AI, according to Terah Lyons, who formerly led emerging technology policy for President Barack Obama, “are human failings.” “If you’re not thinking about the human problem,” she explains, “then AI isn’t going to solve it for you.”
Though AI bias is very real, it’s a fixable problem. Researchers assert that building accountability into AI is not just about computational approaches but looking at the sociological and other parameters behind the ideas and processes that generated these technologies and systems.
AIs reflect biases
The idea that technology has a solution for everything and technological solutions are superior to others is what Meredith Broussard refers to as “technochauvinism.” “AI [and] computers are just machines,” says Broussard, a data journalist and professor at New York University. “And we need to come to grips with the idea that there are a lot of flaws in our computational systems, [that] computers make a lot of biased decisions, and we should not just blindly turn everything over to [them].”
A study by Madalina Vlasceanu and David Amodio, published in July 2022, demonstrates how AIs propagate and reinforce gender bias. The researchers report that internet search algorithms—something most people use multiple times a day—produce biased outputs. When we use these results to inform our everyday behaviors and decisions in real-life situations, they end up reinforcing existing social disparities. Hiring decisions are an example.
In one of their experiments, the researchers showed study participants screenshots of Google image search results of four obscure professions. “What people didn’t know is that we controlled the gender distribution in these screenshots,” Vlasceanu says. “We [then] asked people to judge who is more likely to have each given profession, a man or a woman.”
This last question—about which gender is more likely to have a certain job—was asked twice, once before showing the screenshots and once after.
Before seeing the screenshots, people responded that men are more likely to have all professions. But afterward, “this male bias vanished for the professions which the screenshots showed an equal number of men [and] women,” Vlasceanu adds. “Similarly, when people had to make a hiring choice between a man and a woman for each profession, they chose more men in the professions which the screenshots showed more men performing them, and chose more women [when] screenshots showed an equal gender distribution.”
The researchers also observed that a single exposure to the biased search output was enough to generate correspondingly biased judgments. “[Though] we found this pattern [reflected] in hiring decisions,” Vlasceanu points out, “these threats to equality exist in all areas of society, from education to healthcare allocation.” She and Amodio acknowledge that real-world decisions have intersecting complexities, but their experiment is a valid proof of concept, they contend, demonstrating algorithmic bias propagation.
Machines are easier to ‘forgive’
Research also shows that people are less morally outraged when machines discriminate than when humans do. “People see humans who discriminate as motivated by prejudice, such as racism or sexism, but they see algorithms that discriminate as motivated by data, so they are less morally outraged,” says Yochanan Bigman, a researcher in ethics and human-robot interaction.
Bigman and colleagues wrote a paper about the results. “We found that, systematically, people were less morally outraged when it was an algorithm,” Bigman says. “[They] were still upset, but [less so] when an algorithm did it versus a human.”
This is concerning from a social perspective, he continues. “It means that companies can hide behind an algorithm. Plus, moral outrage is an important societal mechanism to motivate people to address injustices. “If the public is not upset at something, nothing will change,” he says. Plus, it might lead people to believe a stereotype is correct because “it’s in the data,” and data is neutral.
The truth, though, is more complicated.
The roots of algorithmic discrimination lie not in the computational but in the sociological. “An AI-based system is trained on historical data,” Broussard reminds us. “[It] contains all of the existing problems of the world. It reflects systemic racism; it reflects gender discrimination; it reflects caste bias. And so when you train an algorithm and system on data about the world as it is, you are teaching that system to reproduce all of these distinct biases of the world.”
Going beyond the computational
There are methods to mathematically discern the different kinds of bias inside systems, Broussard says, and sometimes people imagine that we can use computers to de-bias systems. “That is not exactly true—there’s pretty much going to be bias in the system, you just have to decide what kind is acceptable.” The question is, who gets to decide that?
One way to check for bias within systems is through algorithmic auditing: “an effort to ensure that the context and purpose surrounding machine learning applications directly inform evaluations of their utility and fairness.” This, for example, can be done with the help of inclusive datasets to test if algorithms and AI models are churning out problematic results before such potentially problematic technologies are widely deployed.
This, though, is by no means a fix-all solution. As researcher Mona Sloane writes in an op-ed, this is because there is no clear definition of what an algorithmic audit is. She suggests three steps as a backdrop to auditing systems: transparency about where and how AI tools are deployed; a clearly defined scope of an “audit” in an automated system; and an understanding of how it can be operationalized.
“It requires you to have really hard conversations and to admit that there is a problem in a computational system,” Broussard says. “We do need to have these hard conversations; we do need to admit flaws and give ourselves opportunities to repair.”
But despite the ongoing work at spaces like NeurIPS, ACM FaCCT, AI Now Institute, Just Data Lab, Algorithmic Justice League, D4BL, and others to articulate and address discrimination by data, “we’re still collectively, though, at the stage of trying to convince people that algorithmic discrimination exists,” Broussard adds.
Better and more even computational literacy, for instance, is needed to make people aware that algorithms discriminate; that computers are not neutral. “But these ideas that computers are objective…are pretty foundational,” she goes on. “It’s something that people have been saying for…the entire digital era. It’s very hard to change that belief, even if it’s wrong, which it is.”
Building a framework for accountable AI needs data scientists, sociologists, psychologists, journalists, artists, ethicists and many others to come together. Psychology itself has a role to play, Vlasceanu says, by revealing, for example, the ways in which people and societies are influenced by these algorithms.
The emerging field of digital humanities—the study of cultural, social and epistemic impacts of digital technologies—also informs understandings of equitable AI. For example, Cambridge Digital Humanities’ AI Forensics project is an attempt “to design a new sociotechnical and political framework for the analysis and critique of visual AI systems.”
As someone who studies moral cognition, Bigman contends that it is important to work together. “Let lawyers be lawyers, legislators be legislators, psychologists be psychologists, and computer scientists be computer scientists… But we each need to understand what we can do.”
The actual hard work of rooting out bias from systems, though, will have to come from humans, not machines. “All of the problems that are easy to solve with technology have been solved,” says Broussard. “So the only problems we’re left with are the really hard ones. And technology is not great at solving social problems. You can’t write an algorithm to erase racism.”
Building an ethics-first framework for AI is a complex and many-layered issue, including pinning down a definition of ethics itself. A report from the Pew Research Center suggests that ethical AI is more than a decade away, but also points out that “no technology endures if it broadly delivers unfair or unwanted outcomes.” A survival-of-the-fittest type of churning is likely to drive out the worst AI technologies, while those that implement “good” AI will be the beacon for the rest of the industry.